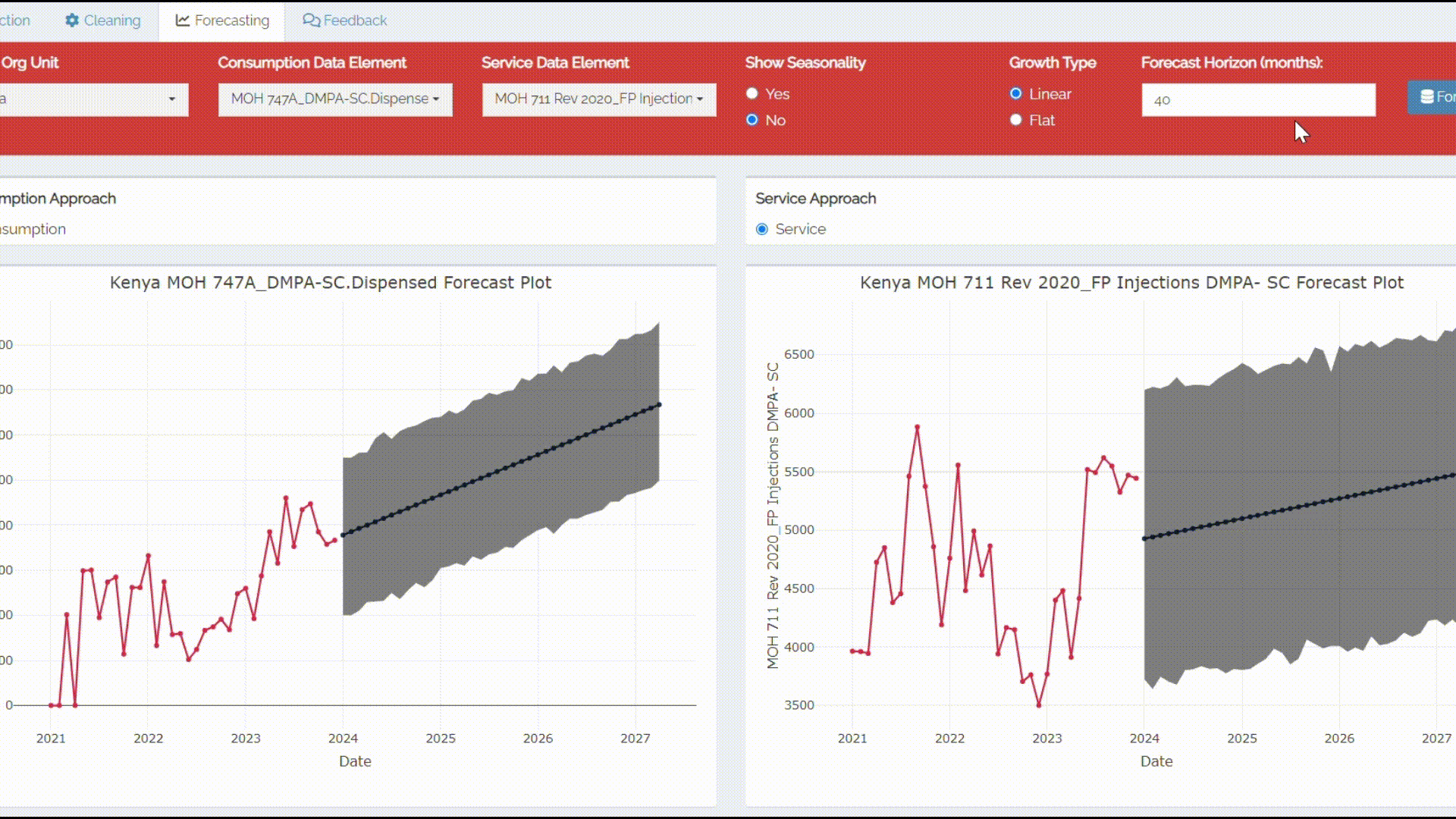
The health sectors in Kenya and Tanzania are undergoing a quiet revolution, transforming how essential medicines are managed and distributed. inSupply Health has pioneered solutions driven by the power of artificial intelligence (AI) to overcome the challenges of timeliness and accuracy in forecasting and supply planning.
Accurate forecasting is undoubtedly the bedrock of effective supply chain management in the health sector. It ensures that the correct number of supplies are available to meet patient needs, reducing costs and preventing shortages and surpluses. Quantification teams in Kenya and Tanzania have faced the challenge of accurately and routinely predicting health commodities requirements for service delivery. Despite strong policies and comprehensive guidance documents, traditional methods of forecasting medicine needs have primarily been manual, time-consuming, and prone to inaccuracies. Specific issues included complex data extraction and organization, extensive data processing, difficulties in identifying usage trends, and the absence of comparative tools. Health workers are already stretched thin and face time-consuming data collection and analysis processes, frequently resulting in errors.
Challenges on the Road to AI Integration: Data, Trust, and Infrastructure
inSupply Health recognized that the key to mitigating these issues lies in developing a roadmap for integrating AI tools into existing systems. In the early stages, skepticism abounded. Many grappled with the idea of relying on algorithms to make decisions that had traditionally been based on human judgment and experience. Moreover, the low quality and availability of data posed significant hurdles. Then, there was the challenge of ensuring that AI tools were not only accurate but also transparent and interpretable. Government partners expressed concerns that using AI for forecasting could lead to potential data security concerns, such as the risk of unauthorized access to sensitive patient information. Healthcare providers also needed to trust that the recommendations made by AI were based on sound reasoning and not just black box algorithms. Building this trust required engaging stakeholders in the development process and using dummy data to demonstrate the models` functionality and value add they bring.
inSupply Health worked with stakeholders in Kenya and Tanzania to create a collaborative environment to successfully implement AI-powered processes and tools. This involved reversing the traditional implementation process by first consulting with the stakeholders to create a tailored solution that met their specific needs rather than developing a solution and then taking it to stakeholders. Using machine learning algorithms, inSupply Health built predictive models that were integrated into existing systems to enable a process where the forecasting of essential medicines could be done with unprecedented accuracy. The approach involved multiple steps and was designed to improve efficiency, data quality, and decision-making processes at all levels of the supply chain. First, opportunities to improve all steps in the quantification process were identified, including data extraction, cleaning, and trends analysis. Then, AI solutions were designed and customized for each opportunity before beta-testing, fine-tuning, and implementation at both the national and subnational levels. The team operated under some general hypotheses, namely:
- Using imperfect data is better than not using any data.
- The more routinely the data is used, the better the opportunity to improve it.
- Technology is likely to be adopted, scaled, and sustained when it solves a real problem defined by users in a way that works for them.
The collaborative approach, which necessitated multiple rounds of consultation, meetings, and enabling users to define requirements iteratively, was time-consuming. However, it helped inSupply Health overcome the challenge of building trust among local stakeholders and obtaining their buy-in and enthusiasm for the AI-powered solutions.
Implementing AI Solutions for Change
In Kenya, forecasting approaches vary significantly across programs. National health programs, including the maternal, newborn, and child health (MNCH) and family planning (FP) programs, used national-level, aggregated data from the Kenya Health Information System (KHIS) to carry out annual quantification. In contrast, data for thousands of essential medicines for primary health care is only captured on stock cards in facilities across the country’s 47 counties. This data was sampled and collected manually, a time consuming and expensive process that understandably limits the frequency of forecasting to periods of funding availability.
In Tanzania, the eLMIS has a Bottom-Up Quantification (BUQ) model within it that generates forecasts using facility-level data that is normally manually entered into it to generate forecasts. However, facilities need to manually review their forecasts for each individual item which ranges from 200-1000 items depending on the facility (dispensary, hospital etc) because the process is tedious, facilities generally do not undertake a thorough review and push data to the district with significant quality issues, with the same reason these forecasts pass the district and regional to the national level which leads to procurement that is based on non accurate forecasts. Stakeholder engagement across all levels ensured the creation of four user-centric AI tools that could be integrated seamlessly into existing systems. Each tool was adapted for the specific context, system, program, and commodity group. In Kenya, three tools covered the FP and MNCH programs and county-specific forecasting needs, while in Tanzania, a replicable model of the BUQ process in the eLMIS was created to pave the way for integration and sustainability if accepted and adopted. While it is yet to be adopted nationwide, this approach has been piloted in one council with promising results, and there are plans for a broader integration into the eLMIS.
inSupply Health worked closely with national and sub-national counterparts at the Ministry of Health (MOH) in both countries to use all four tools during annual forecasting processes. They solicited feedback, taking note of evolving user and process needs, and ensuring each step was well understood and approved by the MOH decision-makers. Examples of user-defined adaptations to the tool included human-in-the-loop touchpoints for users to accept or decline AI outputs, such as using seasonal data and accepting adjusted and cleaned data.
A New Dawn: Benefits of Using AI in Supply Chain Management
The benefits of using AI for forecasting were immediately evident in the significant time savings. The forecasting process that used to take weeks of manual data analysis and painstaking assumption building could now be done in minutes. In Kenya, the data processing time was reduced from nearly three days to 15 minutes, while in Tanzania, it was reduced from three weeks to a single day. The time saving also enabled users to customize the model parameters based on their awareness of the historical data, such as disregarding seasonal predictions for certain commodities, thereby enhancing the decision-making process when selecting forecast outputs. By providing accurate and timely data, the AI tools enabled health officials to make informed decisions about resource allocation and policy planning. This holistic approach is crucial for building a health system that adapts and responds to the ever-changing public health landscape.
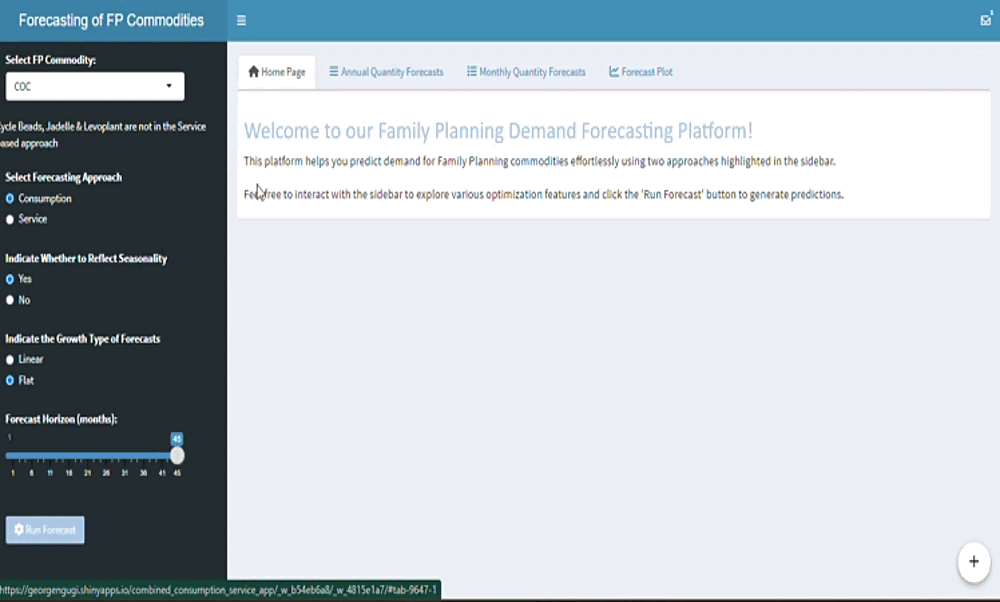
Looking to the Future: Scaling Up AI Innovations
Integrating AI into the supply chain management systems for essential medicines has had a tremendous impact in Kenya and Tanzania, paving the way for improving the availability of life-saving medicines and empowering health care workers. One of the key takeaways from the process is that it is still important to approach forecasting as both an art and a science, especially when combining AI with imperfect and incomplete data to generate estimates. The AI approaches in Kenya and Tanzania continue to evolve and are laying the groundwork for more resilient health systems. By streamlining forecasting and supply planning processes to reduce the risk of stockouts, program leaders are helping to ensure that essential medicines are available and gradually building the foundation for a resilient health system that can adapt and respond to evolving public health challenges.
In highlighting the positive impact of AI solutions in the forecasting process, Dr. Sospeter Gitonga of Kenya’s Division of Health Products and Technologies (DHPT) pointed out that “Quantification of health products and technologies in Kenya has for a long time been a laborious process due to the manual nature of collation and analysis of data.” He added that the use of AI has enhanced the forecasting outputs at the county and national levels, leading to better-informed decisions for budget allocation.
Dennis Kapinga, a Regional Laboratory Specialist in Tanzania’s Coast Region, highlighted the importance of solutions to local challenges developed by local experts, adding that, “the future of the BUQ is promising.”
This underpins another important lesson that the team learned: building trust and ownership is a vital component of the process when co-developing solutions. This can happen when stakeholder engagement is done early and continuously.
As we look to the future, the continued development and scaling up of AI technologies hold the promise of even more significant advancements in health care. The success in Kenya and Tanzania serves as a beacon for other countries grappling with similar challenges, demonstrating that it is possible to transform health systems and save lives with the right tools and partnerships. The journey is far from over, but the progress made so far is a testament to the power of technology and the resilience of those dedicated to improving health outcomes.
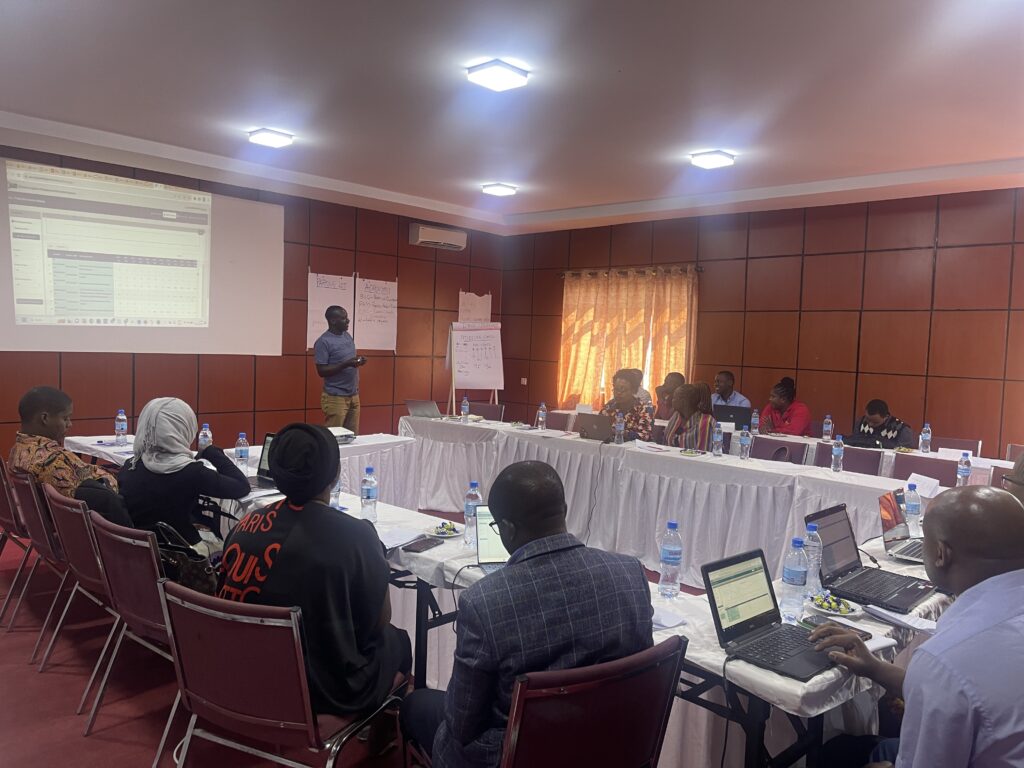